- Functional Genomics Book Pdf
- Bioinformatics And Functional Genomics
- Functional Genomics Methods And Protocols Pdf
- Pevsner Bioinformatics And Functional Genomics Pdf
- Functional Genomics Pdf
3.3 Functional Genomics. Functional genomics is a wide approach for predicting functions and interactions of genes and their products. As described in the previous section, the advancement of genome-sequencing platforms has made it possible to fully sequence a large number of plant genomes. Nov 08, 2012 Introduction. T he field of functional genomics attempts to describe the functions and interactions of genes and proteins by making use of genome-wide approaches, in contrast to the gene-by-gene approach of classical molecular biology techniques. It combines data derived from the various processes related to DNA sequence, gene expression, and protein function, such as coding. Jan 28, 2005 Microbial Functional Genomics offers a timely summary of the principles, approaches, and applications. It presents a comprehensive review of microbial functional genomics, covering microbial diversity, microbial genome sequencing, genomic technologies, genome-wide functional analysis, applied functional genomics, and future directions. Functional Genomics - Lecture 3 Slides borrowed from: Debbie Laudencia-Chingcuanco, USDA-ARS Cheryl Seaton, USDA-ARS Victoria Carrollo, USDA-ARS Zjelka McBride, UC Davis. Welcome to the website for Bioinformatics and Functional Genomics (3rd edition, Wiley-Blackwell, 2015). This site features a complete bioinformatics teaching curriculum: PowerPoints for an entire course taught at the Johns Hopkins School of Medicine, and web site links organized by chapter in the new textbook, Bioinformatics and Functional Genomics.
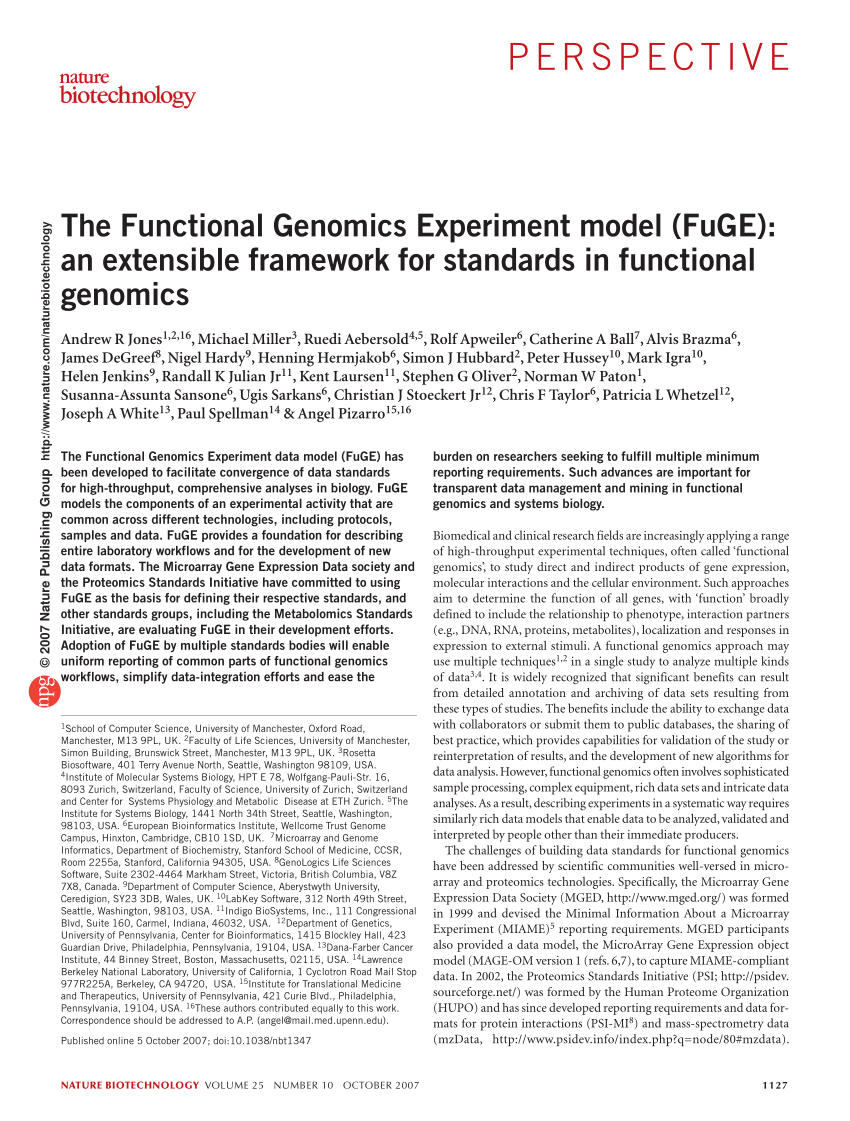
Abstract
Objective
Over the past decade, the development of high-throughput technologies for DNA and protein analysis has revolutionized the ways in which cells can be studied. Within a relatively short time frame, research has changed from studying individual genes and proteins to analyzing entire genomes and proteomes.
Approach
In this article, we summarize the technologies and concepts that form the basis of this functional genomics approach.
Results
Microarray and next-generation sequencing technologies have allowed researchers to investigate many different aspects of the cell, including DNA mutations, histone modifications, DNA methylation, chromatin structure, transcription, and translation on a genome-wide level. In addition, mass spectrometry technologies have undergone significant development and currently enable us to globally profile protein levels, protein–protein interactions, post-translational protein modifications, and metabolites.
Innovation and Conclusion
The integration of information from the various processes that occur within a cell provides a more complete picture of how genes give rise to biological functions, and will ultimately help us to understand the biology of organisms, in both health and disease.
Introduction
The field of functional genomics attempts to describe the functions and interactions of genes and proteins by making use of genome-wide approaches, in contrast to the gene-by-gene approach of classical molecular biology techniques. It combines data derived from the various processes related to DNA sequence, gene expression, and protein function, such as coding and noncoding transcription, protein translation, protein–DNA, protein–RNA, and protein–protein interactions. Together, these data are used to model interactive and dynamic networks that regulate gene expression, cell differentiation, and cell cycle progression.
Studying cells at a systems level has been facilitated by recent technological advancements, as well as the availability of complete genome sequences. Since the landmark publication of the first draft of the human genome in 2001,, the genomes of hundreds of organisms from all branches of the tree of life have been sequenced. This has lead to improved annotations of genes and their products, and has enabled genome-wide studies aimed at understanding interactions and molecular processes in the cell.
Clinical Problems Addressed
This article will give a brief overview of high-throughput omics technologies and their applications, and how these powerful tools have expanded the possibilities for studying the complex biology of cells, organs, and full organisms.
Materials and Methods
DNA microarrays
DNA microarrays consist of thousands of microscopic DNA spots (probes) that are bound to a solid surface, such as glass or a silicon chip (Affymetrix) or microscopic beads (Illumina). Labeled single-stranded DNA or antisense RNA fragments from a sample of interest are hybridized to the DNA microarray under high-stringency conditions. Each probe is identified by its location on the DNA microarray, and the amount of hybridization detected for a specific probe is proportional to the level of nucleic acids from the corresponding genomic location in the original sample.
Next-generation sequencing technologies
Three main next-generation sequencing (NGS) platforms are widely used: the Roche 454 platform (Roche Life Sciences), the Applied Biosystems SOLiD platform (Applied Biosystems), and the Illumina (formerly known as Solexa) Genome Analyzer and HiSeq platforms (Illumina). For these three NGS platforms, template DNA is fragmented, bound to adaptors, amplified by polymerase chain reaction, and subsequently immobilized on beads or on an array where clusters consisting of identical DNA fragments are formed. These clusters are read by sequential cycles of nucleotide incorporation, washing, and detection, where the number of cycles eventually determines the read length (Fig. 1). A fourth DNA sequencing technology has been recently developed by Ion Torrent. The Ion Torrent technology takes advantage of the hydrogen ion that is released as a byproduct of the incorporation of a nucleotide into a DNA strand by polymerase. The sequencer directly senses the ions produced by template-directed DNA polymerase synthesis on a massive parallel semiconductor-sensing device that directly transforms this chemical signal to digital information.
Comparison between Sanger sequencing and next-generation sequencing (NGS) technologies. Sanger sequencing is limited to determining the order of one fragment of DNA per reaction, up to a maximum length of ∼700 bases. NGS platforms can sequence millions of DNA fragments in parallel in one reaction, yielding enormous amounts of data. To see this illustration in color, the reader is referred to the web version of this article at www.liebertpub.com/wound
Over the years, sequencing pipelines have greatly improved in throughput and costs for instruments and reagents, along with improvements in computational power, data storage, and bioinformatics tools that facilitate the analysis of the growing quantities of sequence reads. Together, these advancements have caused a dramatic drop in sequencing costs, down to about $0.09 (U.S.) per megabase in early 2012.7 Several new companies, such as Helicos Biosciences, Pacific Biosciences, and Oxford Nanopore Technologies, are currently developing novel, so-called third generation sequencing techniques that do not require amplification of template DNA, but are able to read the sequence of single DNA molecules., These technologies could significantly advance the sequencing field by greatly reducing the cost for reagents and improving the throughput, while simultaneously eliminating any bias introduced during the template amplification step of the NGS protocol.
Mass spectrometry
A mass spectrometer consists of three components: an ion source to convert a gas-phase sample into ions, a mass analyzer to separate the ions by means of an electromagnetic field, and a detector. The development of ionization techniques that enable the transfer of proteins and peptides into the gas phase without substantial degradation has been crucial for the application of mass spectrometry (MS) in large-scale proteomic studies. The most commonly used ionization techniques are matrix-assisted laser desorption ionization and electrospray ionization. These ionization techniques can be combined with various types of mass analyzers that separate ions based on the mass-to-charge ratio by either trapping ions in an electrical field (trapping mass spectrometers) or by accelerating ions through an electrical field and measuring the time-of-flight. A comparison of instrument configurations that are most commonly used in proteomics is provided elsewhere. The most advanced mass spectrometer available to date is the Orbitrap, which has a high resolution, a high mass accuracy, and a large dynamic range that make it suitable for a wide range of proteomics and metabolomics applications.
The most common strategy for proteomic studies is a bottom-up approach, in which a protein sample is first enzymatically digested into smaller peptides, followed by separation of the peptides by charge, hydrophobicity, or a combination of these characteristics, and then injected into the mass spectrometer. Individual peptide spectra are used to indirectly identify complete proteins that were present in the original sample.
Results
Genomics
For almost 30 years, sequencing of DNA has largely been dependent on the first-generation Sanger dideoxy sequencing method. Sanger sequencing requires each sequence read to be amplified and read individually (Fig. 1). Despite considerable improvements in automation and throughput, Sanger sequencing remains relatively expensive and labor intensive. For whole-genome sequencing, it is dependent upon bacterial cloning, which is time-consuming and can introduce biases as a result of, for example, difficulties in cloning AT-rich fragments or genes that are toxic to bacteria. Since 2005, several NGS technologies have become commercially available, which have transformed the field of whole-genome sequencing. The amount of data generated in parallel from small amounts of DNA is enormous, and currently reaches up to 6 billion short reads or 600 gigabase per instrument run. This has greatly facilitated the sequencing of the complete genome of organisms to identify DNA mutations, ranging from single-nucleotide polymorphisms to large gene deletion or duplication events. In addition, these technologies have enabled a range of novel applications, including genome-wide analysis of epigenetic mechanisms, such as DNA methylation, location of histone modifications, transcription factors binding events, and nucleosome positioning, as well as profiling of gene expression (see Transcriptomics section).
Many of these applications are based on mapping short reads of DNA obtained from a particular sample to a reference genome and analyzing the distribution of these reads (Fig. 2). For example, for determining the locations of a particular histone modification, chromatin is sheared into mononucleosomal fragments. Chromatin fragments containing the histone modification of interest are immunoprecipitated and the corresponding DNA fragments are sequenced (ChIP-Seq). Since only the 5′ end of DNA fragments are sequenced, the sequence reads obtained in this experiment will map to the outer side of the nucleosome. However, the midpoint of the histone can be determined by extrapolation of the distribution of sequence reads from either side of the nucleosome. This type of experimental setup and data analysis yields highly accurate positioning of modified histones. Novel experimental procedures are continuously being applied to achieve even a higher resolution, recently yielding single-base pair resolution for both transcription factor binding sites and nucleosome positioning.
Applications of NGS. The types of experiments that can be performed using NGS are many fold and are certainly not limited to the applications listed here. Applications include sequencing the complete genome or exome (all coding regions of the genome) to identify single-nucleotide polymorphisms (SNP-Seq) or other DNA mutations, profiling the genome-wide locations of methylated cytosines (Bisulfite-Seq), investigating various aspects of chromatin structure and regulation of gene expression by determining nucleosome positioning (MAINE-Seq and FAIRE-Seq), histone modifications or transcription factor binding (ChIP-Seq), and determining mRNA levels to study gene expression and its regulation (RNA-Seq). To see this illustration in color, the reader is referred to the web version of this article at www.liebertpub.com/wound
NGS has greatly improved our ability to study the various genetic and epigenetic mechanisms, with unprecedented detail and specificity. This information has provided us with enormous insight into gene regulation and cell cycle control, as well as the roll of mutations and epigenetic mechanisms in pathogenesis.
Transcriptomics
Regulation of gene expression is fundamentally important for cell development and differentiation. Profiling the abundance of transcripts in different cell types and under various conditions increases our knowledge about gene function and regulatory pathways. In the past, RNA transcripts have been analyzed using Northern blotting or reverse transcription polymerase chain reaction, which are restricted to limited numbers of known transcripts. Serial analysis of gene expression (SAGE) was developed in 1995, and consists of sequencing small tags that correspond to the 3′ fragments of messenger RNA (mRNA). This allows for a highly quantitative analysis by simply counting the number of tags that map to a particular gene. Despite several improvements to the original protocol, SAGE is no longer widely used as it is very labor intensive and relatively low-throughput compared to newly developed NGS applications.
Around the same time, the first DNA microarrays were developed for measuring the expression levels of large numbers of genes., Transcripts isolated from a sample of interest are converted into cDNA or cRNA, are labeled, and are subsequently hybridized to the DNA microarray. The amount of hybridization detected for a specific probe is proportional to the transcript level of the corresponding gene. Comparing transcript levels between various cell types or conditions can be used to identify genes that are involved in cell differentiation or in responses to certain environmental changes. Cluster analysis is often employed to characterize genes that have similar expression profiles and are therefore likely to have similar biological functions. DNA microarrays are still in use today and continue to provide valuable biological information, although it is to be expected that gene expression profiling will shift more and more toward the use of NGS tools.
NGS technologies have opened the door for a broad range of genome-wide analyses related to gene expression and transcript profiles, which are collectively known as RNA-Seq (Fig. 2). Sequence reads derived from an RNA sample of interest are mapped to a reference genome, where the number of reads that map to a certain gene corresponds to the expression level of that gene. Besides profiling gene expression levels, RNA-Seq can be used to analyze transcript boundaries and intron/exon junctions and to discover novel transcripts and novel alternative splice variants. In addition, it can be applied to, for example, profiling of noncoding RNA, nascent transcripts, and ribosome-associated mRNA, and has the potential to immensely increase our understanding of the different roles of RNA and of the various levels of regulation of gene expression. RNA-Seq provides a combination of high-throughput, large sequencing depth, and genome-wide coverage, which is not offered by any other tool used for gene expression analysis in the past. An additional advantage of RNA-Seq over DNA microarrays is that it is not dependent on the availability of a microarray for the species of interest and can therefore be implemented for all organisms.
Proteomics
Proteins are one of the functional units of the cell, and it is therefore essential to understand how proteins function to completely understand biological processes. Since transcript levels do not necessarily correlate with protein levels, quantitation of proteins is required to unequivocally determine their abundance. In addition, many proteins are post-translationally modified, adding an extra level of complexity to their structure and function.
Analysis of the protein content of cells can be performed by two-dimensional gel electrophoresis, where proteins are separated first by size, and then by charge, followed by MS. Another relatively straightforward proteomics approach is geLC-MS, where proteins are first separated by one-dimensional gel electrophoresis (SDS-PAGE). Each gel lane is then divided into equally sized sections, and the proteins from each section are digested, separated by liquid chromatography, and analyzed by MS. More recently, a high-throughput technology has been developed that is more suitable for identifying large numbers of proteins from complex mixtures. Using the multidimensional protein identification technology (MudPIT), proteins are digested into peptides that are then separated by means of two-dimensional chromatography, based on both charge and hydrophobicity, and are subsequently analyzed by MS. The signals of each peptide obtained using MS can then be compared to a database of previously sequenced proteins or to a database of predicted proteins based on the genome sequence to identify the protein from which the peptide was derived. MudPIT allows for a highly sensitive detection of proteins and has over the last decade been applied to a broad range of cells and organisms. It has successfully been used to profile organelle and membrane proteins, identify post-translational modifications, dissect protein complexes, and analyze protein expression.
Interactomics
Determining the abundance and localization of a protein is not sufficient to understand its function. Many molecular processes in the cell are performed by complexes of proteins that are organized by protein–protein interactions. Such functional interactions are found in signal transduction, transcriptional regulation, metabolic pathways, and many other biological functions. Deciphering these interactions is crucial to understanding the interactive pathways and networks that form the basis of many cellular processes.
Protein–protein interactions can be studied using a variety of methods. The two-hybrid system has been used for the first time in 19897 and has since then been modified to allow proteome-scale screening., In the two-hybrid method, one protein of interest is fused to a DNA binding domain, while another protein of interest is fused to an activation domain. Both fusion proteins are then expressed in the same cell, which could in theory be any living cell, although yeast and bacterial cells are most widely used. If the proteins interact, a reporter gene is transcriptionally activated, which will change the phenotype of the cell and allow for an easy readout. In addition to the original two-hybrid system, which requires proteins to be present in the nucleus, the cytotrap yeast two-hybrid tool has been developed for detection of protein–protein interactions in the cytoplasm. The two-hybrid technique is relatively straightforward and can be used as a first screen to identify interacting protein partners. However, the rate of false positives is relatively high, and interactions found by the two-hybrid technique should always be validated using other tools.
While the two-hybrid system is limited to screening the interaction between two proteins at a time, affinity purification methods may be more suitable to study the organization of proteins into complexes. This technique entails fusing a tag to a protein of interest, which is subsequently used to isolate this protein together with all proteins that are bound. The bound proteins are then analyzed by MS. In 2002, this technique was first performed in yeast and revealed thousands of protein–protein interactions, many of which had not been described before., Since then, the tandem affinity purification (TAP) strategy has become increasingly popular. TAP involves two rounds of affinity purification that provide a high specificity, but may on the other hand result in the loss of transient or very weak protein–protein interactions. In combination with other tools, such as protein microarray and phage display, these technologies have vastly increased our understanding of interactive protein networks.
Metabolomics
Metabolites are small molecules, such as amino acids, sugars, and fatty acids, that are chemically transformed by enzymes during metabolism and that play critical roles in various biological processes. Metabolite levels correlate more directly with a cellular phenotype than genes or proteins, and therefore provide an accurate functional readout of the state of a cell. Researchers have long been interested in profiling metabolites on a global level, but only recently technologies have emerged that enable these types of studies. The tools most widely used for global metabolomics approaches are nuclear magnetic resonance (NMR) and liquid chromatography coupled to MS. The main advantages of NMR are its high reproducibility and ease of sample preparation. However, the sensitivity of MS-based techniques is higher compared to NMR, and allows the detection of most metabolites present in a cell. Information from metabolomics studies will increase our understanding of complex cellular metabolism, characterize new metabolic pathways, and identify new targets for therapeutic intervention in, for example, cancer.
Discussion
With a plethora of information emerging from various omics studies, the main challenge in systems biology is to integrate these data into a single network and to find out how genes, transcripts, proteins, and metabolites interact to regulate the biological processes that determine cell function and cell cycle progression (Fig. 3). The availability of large amounts of data has led to the development of more robust computational methods for network analysis. These tools can, for example, be used to predict protein function by means of guilt-by-association analysis. This type of analysis is based on the principle that the function of a protein is likely to resemble the function of proteins with which it interacts or is coexpressed. In addition, multiple tools are available that support pathway analyses to determine whether certain pathways or gene ontologies are over-represented in certain biological processes.
Schematic overview of network analysis. Integration of information from different aspects of the cell, such as genome, transcriptome, proteome, interactome, and metabolome, will increase our understanding of how these components are interconnected and how these interactions determine biological functions. To see this illustration in color, the reader is referred to the web version of this article at www.liebertpub.com/wound
To obtain accurate and complete cell models, network analysis should not only be based on experiments performed in model organisms under standard laboratory conditions. In contrast, using information obtained from multiple species, cell types, or under various environmental conditions will allow differentiation between relatively static housekeeping genes and the dynamic processes involved in response to stress or other external and internal signals. This will ultimately lead to building improved models of biologically relevant interactions between all components of a cell.
Innovation
Novel technologies developed over the past decade allow a systems biology approach to studying the complex processes that shape cells, organs, and organisms. Instead of focusing on single genes or proteins, NGS platforms and MS applications provide the opportunity to study genes, transcripts, proteins, and their interactions on a genome-wide level. Ultimately, the integration of this information will result in an improved understanding of how genes give rise to biological functions.
NGS technologies enable a range of applications for studying various cellular processes related to DNA, chromatin structure, transcription, and translation on a genome-wide level.
Advances in MS allow large-scale studies into proteins, protein–protein interactions, post-translational protein modifications, and metabolites.
Integration of genome-wide data by network analyses will improve our understanding of cellular biology.
Abbreviations and Acronyms
AT | adenine+thymine |
cDNA | complementary deoxyribonucleic acid |
ChIP-Seq | chromatin immunoprecipitation coupled to next-generation sequencing |
cRNA | complementary ribonucleic acid |
ddNTP | dideoxy nucleotide triphosphate |
DNA | deoxyribonucleic acid |
FAIRE | formaldehyde-assisted isolation of regulatory elements |
GeLC-MS | gel electrophoresis coupled to liquid chromatography and mass spectrometry |
MAINE | micrococcal nuclease-assisted isolation of nucleosomes |
mRNA | messenger ribonucleic acid |
MS | mass spectrometry |
MudPIT | multidimensional protein identification technology |
NGS | next-generation sequencing |
NMR | nuclear magnetic resonance |
RNA | ribonucleic acid |
RNA-Seq | high-throughput ribonucleic acid sequencing |
SAGE | serial analysis of gene expression |
SDS-PAGE | sodium dodecyl sulfate–polyacrylamide gel electrophoresis |
TAP | tandem affinity purification |
Acknowledgments and Funding Sources
E.M.B. is supported by the Human Frontier Science Program (grant LT00507/2011-L) and K.G.R. is supported by the National Institutes of Health (grant R01 AI85077-01A1).
Author Disclosure and Ghostwriting
No competing financial interests exist. The content of this article was expressly written by the authors listed. No ghostwriters were used to write this article.
Functional Genomics Book Pdf
About The Authors
Dr. Evelien Bunnik is a post-doctoral fellow and Dr. Karine Le Roch is an associate professor at the University of California, Riverside, CA. They use functional genomics approaches, such as proteomics and high-throughput sequencing technologies to elucidate critical regulatory networks driving the malaria parasite life cycle progression and to identify novel drug targets.
References
Functional genomics is a field of molecular biology that attempts to describe gene (and protein) functions and interactions. Functional genomics make use of the vast data generated by genomic and transcriptomic projects (such as genome sequencing projects and RNA sequencing). Functional genomics focuses on the dynamic aspects such as gene transcription, translation, regulation of gene expression and protein–protein interactions, as opposed to the static aspects of the genomic information such as DNA sequence or structures. A key characteristic of functional genomics studies is their genome-wide approach to these questions, generally involving high-throughput methods rather than a more traditional “gene-by-gene” approach.
- 2Techniques and applications
- 2.1At the DNA level
- 2.2At the RNA level
- 2.3At the protein level
- 2.4Loss-of-function techniques
- 2.5Functional annotations for genes
- 4Consortium projects focused on Functional Genomics
Definition and goals of functional genomics[edit]
In order to understand functional genomics it is important to first define function. In their paper[1] Graur et al. define function in two possible ways. These are 'Selected effect' and 'Causal Role'. The 'Selected Effect' function refers to the function for which a trait(DNA, RNA, protein etc.) is selected for. The 'Causal role' function refers to the function that a trait is sufficient and necessary for. Functional genomics usually tests the 'Causal role' definition of function.
The goal of functional genomics is to understand the function of genes or proteins, eventually all components of a genome. The term functional genomics is often used to refer to the many technical approaches to study an organism's genes and proteins, including the 'biochemical, cellular, and/or physiological properties of each and every gene product'[2] while some authors include the study of nongenic elements in their definition.[3] Functional genomics may also include studies of natural genetic variationover time (such as an organism's development) or space (such as its body regions), as well as functional disruptions such as mutations.
The promise of functional genomics is to generate and synthesize genomic and proteomic knowledge into an understanding of the dynamic properties of an organism. This could potentially provide a more complete picture of how the genome specifies function compared to studies of single genes. Integration of functional genomics data is often a part of systems biology approaches.
Techniques and applications[edit]
Functional genomics includes function-related aspects of the genome itself such as mutation and polymorphism (such as single nucleotide polymorphism (SNP) analysis), as well as the measurement of molecular activities. The latter comprise a number of '-omics' such as transcriptomics (gene expression), proteomics (protein production), and metabolomics. Functional genomics uses mostly multiplex techniques to measure the abundance of many or all gene products such as mRNAs or proteins within a biological sample. A more focused functional genomics approach might test the function of all variants of one gene and quantify the effects of mutants by using sequencing as a readout of activity. Together these measurement modalities endeavor to quantitate the various biological processes and improve our understanding of gene and protein functions and interactions.
At the DNA level[edit]
Genetic interaction mapping[edit]
Systematic pairwise deletion of genes or inhibition of gene expression can be used to identify genes with related function, even if they do not interact physically. Epistasis refers to the fact that effects for two different gene knockouts may not be additive; that is, the phenotype that results when two genes are inhibited may be different from the sum of the effects of single knockouts.
DNA/Protein interactions[edit]
Proteins formed by the translation of the mRNA (messenger RNA, a coded information from DNA for protein synthesis) play a major role in regulating gene expression. To understand how they regulate gene expression it is necessary to identify DNA sequences that they interact with. Techniques have been developed to identify sites of DNA-protein interactions. These include Chip-sequencing, CUT&RUN sequencing and Calling Cards. [4]
DNA accessibility assays[edit]
Assays have been developed to identify regions of the genome that are accessible. These regions of open chromatin are candidate regulatory regions. Theseassays include ATAC-seq, DNase-Seq and FAIRE-Seq.
At the RNA level[edit]
Bioinformatics And Functional Genomics
Microarrays[edit]
Microarrays measure the amount of mRNA in a sample that corresponds to a given gene or probe DNA sequence. Probe sequences are immobilized on a solid surface and allowed to hybridize with fluorescently labeled “target” mRNA. The intensity of fluorescence of a spot is proportional to the amount of target sequence that has hybridized to that spot, and therefore to the abundance of that mRNA sequence in the sample. Microarrays allow for identification of candidate genes involved in a given process based on variation between transcript levels for different conditions and shared expression patterns with genes of known function.
SAGE[edit]
Serial analysis of gene expression (SAGE) is an alternate method of analysis based on RNA sequencing rather than hybridization. SAGE relies on the sequencing of 10–17 base pair tags which are unique to each gene. These tags are produced from poly-A mRNA and ligated end-to-end before sequencing. SAGE gives an unbiased measurement of the number of transcripts per cell, since it does not depend on prior knowledge of what transcripts to study (as microarrays do).
RNA sequencing[edit]
RNA sequencing has taken over microarray and SAGE technology in recent years, as noted in 2016, and has become the most efficient way to study transcription and gene expression. This is typically done by next-generation sequencing.[5]
A subset of sequenced RNAs are small RNAs, a class of non-coding RNA molecules that are key regulators of transcriptional and post-transcriptional gene silencing, or RNA silencing. Next generation sequencing is the gold standard tool for non-coding RNA discovery, profiling and expression analysis.
Massively Parallel Reporter Assays (MPRAs)[edit]
Massively parallel reporter assays is a technology to test the cis-regulatory activity of DNA sequences.[6][7] MPRAs use a plasmid with a synthetic cis-regulatory element upstream of a promoter driving a synthetic gene such as Green Fluorescent Protein. A library of cis-regulatory elements is usually tested using MPRAs, a library can contain from hundreds to thousands of cis-regulatory elements. The cis-regulatory activity of the elements is assayed by using the downstream reporter activity. The activity of all the library members is assayed in parallel using barcodes for each cis-regulatory element. One limitation of MPRAs is that the activity is assayed on a plasmid and may not capture all aspects of gene regulation observed in the genome.
STARR-seq[edit]
STARR-seq is a technique similar to MPRAs to assay enhancer activity of randomly sheared genomic fragments. In the original publication,[8] randomly sheared fragments of the Drosophila genome were placed downstream of a minimal promoter. Candidate enhancers amongst the randomly sheared fragments will transcribe themselves using the minimal promoter. By using sequencing as a readout and controlling for input amounts of each sequence the strength of putative enhancers are assayed by this method.
Perturb-seq[edit]
Perturb-seq couples CRISPR mediated gene knockdowns with single-cell gene expression. Linear models are used to calculate the effect of the knockdown of a single gene on the expression of multiple genes.
At the protein level[edit]
Yeast two-hybrid system[edit]
A yeast two-hybrid screening (Y2H) tests a 'bait' protein against many potential interacting proteins ('prey') to identify physical protein–protein interactions. This system is based on a transcription factor, originally GAL4,[9] whose separate DNA-binding and transcription activation domains are both required in order for the protein to cause transcription of a reporter gene. In a Y2H screen, the 'bait' protein is fused to the binding domain of GAL4, and a library of potential 'prey' (interacting) proteins is recombinantly expressed in a vector with the activation domain. In vivo interaction of bait and prey proteins in a yeast cell brings the activation and binding domains of GAL4 close enough together to result in expression of a reporter gene. It is also possible to systematically test a library of bait proteins against a library of prey proteins to identify all possible interactions in a cell.
Functional Genomics Methods And Protocols Pdf
AP/MS[edit]
Affinity purification and mass spectrometry (AP/MS) is able to identify proteins that interact with one another in complexes. Complexes of proteins are allowed to form around a particular “bait” protein. The bait protein is identified using an antibody or a recombinant tag which allows it to be extracted along with any proteins that have formed a complex with it. The proteins are then digested into short peptide fragments and mass spectrometry is used to identify the proteins based on the mass-to-charge ratios of those fragments.
Deep Mutational Scanning[edit]
In Deep mutational scanning every possible amino acid change in a given protein is first synthesized. The activity of each of these protein variants is assayed in parallel using barcodes for each variant. By comparing the activity to the wild-type protein, the effect of each mutation is identified. While it is possible to assay every possible single amino-acid change due to combinatorics two or more concurrent mutations are hard to test. Deep Mutational scanning experiments have also been used to infer protein structure and protein-protein interactions.
Loss-of-function techniques[edit]
Mutagenesis[edit]

Gene function can be investigated by systematically “knocking out” genes one by one. This is done by either deletion or disruption of function (such as by insertional mutagenesis) and the resulting organisms are screened for phenotypes that provide clues to the function of the disrupted gene.
Pevsner Bioinformatics And Functional Genomics Pdf
RNAi[edit]
RNA interference (RNAi) methods can be used to transiently silence or knock down gene expression using ~20 base-pair double-stranded RNA typically delivered by transfection of synthetic ~20-mer short-interfering RNA molecules (siRNAs) or by virally encoded short-hairpin RNAs (shRNAs). RNAi screens, typically performed in cell culture-based assays or experimental organisms (such as C. elegans) can be used to systematically disrupt nearly every gene in a genome or subsets of genes (sub-genomes); possible functions of disrupted genes can be assigned based on observed phenotypes.
CRISPR screens[edit]
CRISPR-Cas9 has been used to delete genes in a multiplexed manner in cell-lines. Quantifying the amount of guide-RNAs for each gene before and after the experiment can point towards essential genes. If a guide-RNA disrupts an essential gene it will lead to the loss of that cell and hence there will be a depletion of that particular guide-RNA after the screen. In a recent CRISPR-cas9 experiment in mammalian cell-lines, around 2000 genes were found to be essential in multiple cell-lines.[11][12] Some of these genes were essential in only one cell-line. Most of genes are part of multi-protein complexes. This approach can be used to identify synthetic lethality by using the appropriate genetic background. CRISPRi and CRISPRa enable loss-of-function and gain-of-function screens in a similar manner. CRISPRi identified ~2100 essential genes in the K562 cell-line.[13][14] CRISPR deletion screens have also been used to identify potential regulatory elements of a gene. For example, a technique called ScanDel was published which attempted this approach. The authors deleted regions outside a gene of interest(HPRT1 involved in a Mendelian disorder) in an attempt to identify regulatory elements of this gene[15]. Gassperini et al. did not identify any distal regulatory elements for HPRT1 using this approach, however such approaches can be extended to other genes of interest.
Functional annotations for genes[edit]
Genome annotation[edit]
Putative genes can be identified by scanning a genome for regions likely to encode proteins, based on characteristics such as long open reading frames, transcriptional initiation sequences, and polyadenylation sites. A sequence identified as a putative gene must be confirmed by further evidence, such as similarity to cDNA or EST sequences from the same organism, similarity of the predicted protein sequence to known proteins, association with promoter sequences, or evidence that mutating the sequence produces an observable phenotype.
Rosetta stone approach[edit]
The Rosetta stone approach is a computational method for de-novo protein function prediction. It is based on the hypothesis that some proteins involved in a given physiological process may exist as two separate genes in one organism and as a single gene in another. Genomes are scanned for sequences that are independent in one organism and in a single open reading frame in another. If two genes have fused, it is predicted that they have similar biological functions that make such co-regulation advantageous.
Bioinformatics methods for Functional genomics[edit]
Because of the large quantity of data produced by these techniques and the desire to find biologically meaningful patterns, bioinformatics is crucial to analysis of functional genomics data. Examples of techniques in this class are data clustering or principal component analysis for unsupervised machine learning (class detection) as well as artificial neural networks or support vector machines for supervised machine learning (class prediction, classification). Functional enrichment analysis is used to determine the extent of over- or under-expression (positive- or negative- regulators in case of RNAi screens) of functional categories relative to a background sets. Gene ontology based enrichment analysis are provided by DAVID and gene set enrichment analysis (GSEA),[16] pathway based analysis by Ingenuity [17] and Pathway studio[18] and protein complex based analysis by COMPLEAT.[19]
New computational methods have been developed for understanding the results of a deep mutational scanning experiment. 'phydms' compares the result of a deep mutational scanning experiment to a phylogenetic tree.[20] This allows the user to infer if the selection process in nature applies similar constraints on a protein as the results of the deep mutational scan indicate. This may allow an experimenter to choose between different experimental conditions based on how well they reflect nature. Deep mutational scanning has also been used to infer protein-protein interactions.[21] The authors used a thermodynamic model to predict the effects of mutations in different parts of a dimer. Deep mutational structure can also be used to infer protein structure. Strong positive epistasis between two mutations in a deep mutational scan can be indicative of two parts of the protein that are close to each other in 3-D space. This information can then be used to infer protein structure. A proof of principle of this approach was shown by two groups using the protein GB1.[22][23]
Results from MPRA experiments have required machine learning approaches to interpret the data. A gapped k-mer SVM model has been used to infer the kmers that are enriched within cis-regulatory sequences with high activity compared to sequences with lower activity.[24] These models provide high predictive power. Deep learning and random forest approaches have also been used to interpret the results of these high-dimensional experiments.[25] These models are beginning to help develop a better understanding of non-coding DNA function towards gene-regulation.
Functional Genomics Pdf
Consortium projects focused on Functional Genomics[edit]
The ENCODE project[edit]
The ENCODE (Encyclopedia of DNA elements) project is an in-depth analysis of the human genome whose goal is to identify all the functional elements of genomic DNA, in both coding and noncoding regions. Important results include evidence from genomic tiling arrays that most nucleotides are transcribed as coding transcripts, noncoding RNAs, or random transcripts, the discovery of additional transcriptional regulatory sites, further elucidation of chromatin-modifying mechanisms.
The Genotype-Tissue Expression (GTEx) project[edit]
The GTEx project is a human genetics project aimed at understanding the role of genetic variation in shaping variation in the transcriptome across tissues. The project has collected a variety of tissue samples (> 50 different tissues) from more than 700 post-mortem donors. This has resulted in the collection of >11,000 samples. GTEx has helped understand the tissue-sharing and tissue-specificity of EQTLs.[26]
See also[edit]
References[edit]
- ^Graur D, Zheng Y, Price N, Azevedo RB, Zufall RA, Elhaik E (20 February 2013). 'On the immortality of television sets: 'function' in the human genome according to the evolution-free gospel of ENCODE'. Genome Biology and Evolution. 5 (3): 578–90. doi:10.1093/gbe/evt028. PMC3622293. PMID23431001.
- ^Gibson G, Muse SV. A primer of genome science (3rd ed.). Sunderland, MA: Sinauer Associates.
- ^Pevsner J (2009). Bioinformatics and functional genomics (2nd ed.). Hoboken, NJ: Wiley-Blackwell.
- ^Wang H, Mayhew D, Chen X, Johnston M, Mitra RD (May 2011). 'Calling Cards enable multiplexed identification of the genomic targets of DNA-binding proteins'. Genome Research. 21 (5): 748–55. doi:10.1101/gr.114850.110. PMC3083092. PMID21471402.
- ^Hrdlickova R, Toloue M, Tian B (January 2017). 'RNA-Seq methods for transcriptome analysis'. Wiley Interdisciplinary Reviews. RNA. 8 (1): e1364. doi:10.1002/wrna.1364. PMC5717752. PMID27198714.
- ^Kwasnieski JC, Fiore C, Chaudhari HG, Cohen BA (October 2014). 'High-throughput functional testing of ENCODE segmentation predictions'. Genome Research. 24 (10): 1595–602. doi:10.1101/gr.173518.114. PMC4199366. PMID25035418.
- ^Patwardhan RP, Hiatt JB, Witten DM, Kim MJ, Smith RP, May D, et al. (February 2012). 'Massively parallel functional dissection of mammalian enhancers in vivo'. Nature Biotechnology. 30 (3): 265–70. doi:10.1038/nbt.2136. PMC3402344. PMID22371081.
- ^Arnold CD, Gerlach D, Stelzer C, Boryń ŁM, Rath M, Stark A (March 2013). 'Genome-wide quantitative enhancer activity maps identified by STARR-seq'. Science. 339 (6123): 1074–7. Bibcode:2013Sci...339.1074A. doi:10.1126/science.1232542. PMID23328393.
- ^Fields S, Song O (July 1989). 'A novel genetic system to detect protein-protein interactions'. Nature. 340 (6230): 245–6. Bibcode:1989Natur.340..245F. doi:10.1038/340245a0. PMID2547163.
- ^Tian S, Muneeruddin K, Choi MY, Tao L, Bhuiyan RH, Ohmi Y, Furukawa K, Furukawa K, Boland S, Shaffer SA, Adam RM, Dong M (27 November 2018). 'Genome-wide CRISPR screens for Shiga toxins and ricin reveal Golgi proteins critical for glycosylation'. PLoS Biology. 16 (11). e2006951. doi:10.1371/journal.pbio.2006951.
- ^Hart T, Chandrashekhar M, Aregger M, Steinhart Z, Brown KR, MacLeod G, et al. (December 2015). 'High-Resolution CRISPR Screens Reveal Fitness Genes and Genotype-Specific Cancer Liabilities'. Cell. 163 (6): 1515–26. doi:10.1016/j.cell.2015.11.015. PMID26627737.
- ^Shalem O, Sanjana NE, Hartenian E, Shi X, Scott DA, Mikkelson T, et al. (January 2014). 'Genome-scale CRISPR-Cas9 knockout screening in human cells'. Science. 343 (6166): 84–87. Bibcode:2014Sci...343...84S. doi:10.1126/science.1247005. PMC4089965. PMID24336571.
- ^Gilbert LA, Horlbeck MA, Adamson B, Villalta JE, Chen Y, Whitehead EH, et al. (October 2014). 'Genome-Scale CRISPR-Mediated Control of Gene Repression and Activation'. Cell. 159 (3): 647–61. doi:10.1016/j.cell.2014.09.029. PMC4253859. PMID25307932.
- ^Horlbeck MA, Gilbert LA, Villalta JE, Adamson B, Pak RA, Chen Y, et al. (September 2016). 'Compact and highly active next-generation libraries for CRISPR-mediated gene repression and activation'. eLife. 5. doi:10.7554/eLife.19760. PMC5094855. PMID27661255.
- ^Gasperini, Molly; Findlay, Gregory M.; McKenna, Aaron; Milbank, Jennifer H.; Lee, Choli; Zhang, Melissa D.; Cusanovich, Darren A.; Shendure, Jay (August 2017). 'CRISPR/Cas9-Mediated Scanning for Regulatory Elements Required for HPRT1 Expression via Thousands of Large, Programmed Genomic Deletions'. The American Journal of Human Genetics. 101 (2): 192–205. doi:10.1016/j.ajhg.2017.06.010. PMC5544381.
- ^Subramanian A, Tamayo P, Mootha VK, Mukherjee S, Ebert BL, Gillette MA, et al. (October 2005). 'Gene set enrichment analysis: a knowledge-based approach for interpreting genome-wide expression profiles'. Proceedings of the National Academy of Sciences of the United States of America. 102 (43): 15545–50. Bibcode:2005PNAS..10215545S. doi:10.1073/pnas.0506580102. PMC1239896. PMID16199517.
- ^'Ingenuity Systems'. Archived from the original on 1999-01-25. Retrieved 2007-12-31.
- ^'Ariadne Genomics: Pathway Studio'. Retrieved 2007-12-31.
- ^Vinayagam A, Hu Y, Kulkarni M, Roesel C, Sopko R, Mohr SE, Perrimon N (February 2013). 'Protein complex-based analysis framework for high-throughput data sets'. Science Signaling. 6 (264): rs5. doi:10.1126/scisignal.2003629. PMC3756668. PMID23443684.
- ^Hilton SK, Doud MB, Bloom JD (2017). 'phydms: software for phylogenetic analyses informed by deep mutational scanning'. PeerJ. 5: e3657. doi:10.7717/peerj.3657. PMC5541924. PMID28785526.
- ^Diss G, Lehner B (April 2018). 'The genetic landscape of a physical interaction'. eLife. 7. doi:10.7554/eLife.32472. PMC5896888. PMID29638215.
- ^Schmiedel, Jörn M.; Lehner, Ben (17 June 2019). 'Determining protein structures using deep mutagenesis'. Nature Genetics. doi:10.1038/s41588-019-0431-x.
- ^Rollins, Nathan J.; Brock, Kelly P.; Poelwijk, Frank J.; Stiffler, Michael A.; Gauthier, Nicholas P.; Sander, Chris; Marks, Debora S. (17 June 2019). 'Inferring protein 3D structure from deep mutation scans'. Nature Genetics. doi:10.1038/s41588-019-0432-9.
- ^Ghandi M, Lee D, Mohammad-Noori M, Beer MA (July 2014). 'Enhanced regulatory sequence prediction using gapped k-mer features'. PLoS Computational Biology. 10 (7): e1003711. Bibcode:2014PLSCB..10E3711G. doi:10.1371/journal.pcbi.1003711. PMC4102394. PMID25033408.
- ^Li Y, Shi W, Wasserman WW (May 2018). 'Genome-wide prediction of cis-regulatory regions using supervised deep learning methods'. BMC Bioinformatics. 19 (1): 202. doi:10.1186/s12859-018-2187-1. PMC5984344. PMID29855387.
- ^'Genetic effects on gene expression across human tissues'(PDF). Nature. 550 (7675): 204–213. 12 October 2017. doi:10.1038/nature24277.
External links[edit]
- MUGEN NoE — Integrated Functional Genomics in Mutant Mouse Models
- Bioinformatics and functional genomics — Companion site for Bioinformatics and functional genomics, 2nd ed.